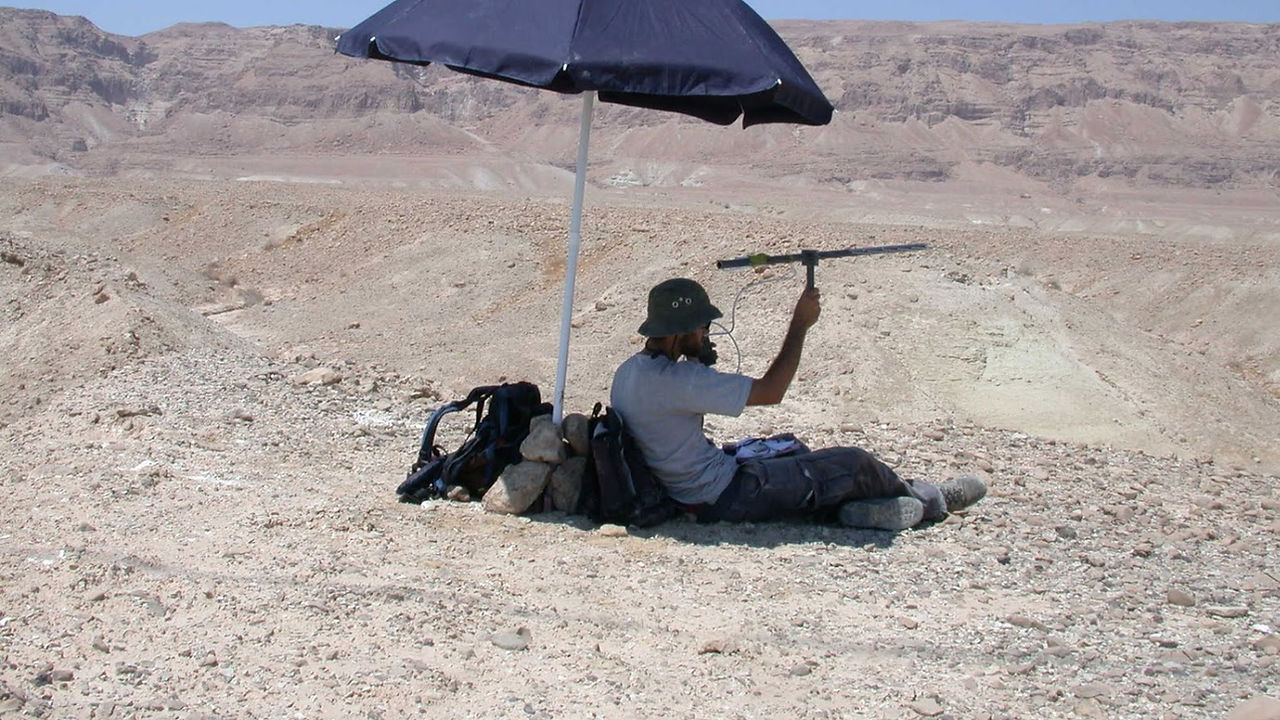
Reviewing papers by others is a part of the game, checkout Orr's Publons profile for contributions as a reviewer or the Research Gate and Google Scholar profiles for publication list
Reviewing papers by others is a part of the game, checkout Orr's Publons profile for contributions as a reviewer or the Research Gate and Google Scholar profiles for publication list
Movement Ecology and Individual Behavior
The challenge: Understanding how animals interact with their physical and social environment is a major question in ecology with implications for conservation and management. However, since their behavior and movement are typically a product of many interacting factors that cannot be easily replicated in small scale experiments, separating between these factors is often challenging. In the context of social interactions, observed interaction rates may reflect social behavior – preferences or avoidance of conspecifics or certain individuals (or phenotypes). Yet, environmental spatiotemporal heterogeneity also affects individual space use and interaction rates. For instance, clumped and ephemeral resources may force individuals to aggregate independently of sociality (i.e., did they meet because the wanted, or simply because they independently came to drink at this place? Do they prefer to meet with this Behavioral type or are they simply tending to use the same resources and coincidentally run into each other?).
The generality: In free ranging animals, social networks are often inferred from spatial proximity. Such proximity-based social networks (PBSNs) are becoming increasingly popular for studying social structures thanks to the parallel improvement of bio-tracking technologies (e.g. GPS and RFIDs) and network randomization methods that allow testing hypotheses on such data. While many of the current methods focus on swapping individual identities among network nodes or in the data streams that underlies the network (e.g. individuals movement paths), we still need better tools to distinguish between the contribution of sociality and other factors towards those interactions (i.e., testing if what we see is different from random really depends how random is defined; does it account for where resources are?).
Our approach: In a recent publication we proposed a novel method that randomizes path segments among different time stamps within each individual separately (Part I). Temporal randomization of whole path segments (e.g. full days) retains their original spatial structure while decoupling synchronization among individuals. This allows researchers to compare observed dyadic association rates with those expected by chance given explicit space use of the individuals in each dyad (i.e., asking are two lizards visit that place together more than they would have if they were visiting it independently of each other?). Further, since environmental changes are commonly much slower than the duration of social interactions, we can differentiate between these two factors (Part II). First, an individual’s path is divided into successive time windows (e.g. weeks), and days are randomized within each time window. Then, by exploring how the deviations between randomized and observed networks change as a function of time window length, we can refine our null model to account also for temporal changes in the activity areas (e.g., accounting for seasonality- all deer in this area have to be in the valleys during winter since this is where resources are, so no wonder they meet more often during winter, but do the meet more that we would expect?).
We have used computer simulations (biased-correlated random walk models) and to validate the method and applied it to a data set of GPS-tracked sleepy lizards (Tiliqua rugosa), demonstrating its ability to reveal the social organization in free-ranging animals while accounting for confounding factors of environmental spatiotemporal heterogeneity.
The next step: should be to broaden the method to identify preferential phenotypic assortment within social network (One prefers to interact with XY and Z on top and beyond the confounding factors that force it to interact with them). And in addition to apply the method to relevant dataset from various taxa and see the generality of emerging ecological patterns.
Its a data analysis challenge, but should be exciting!